A series of controversies surrounding the outcomes of GenAI models has increased advocacy for ethical oversight and governance of AI. While explicit bias, violence, and discrimination have advanced, implicit bias and microaggressions have not.
On the eve of Pride Month 2024, Artefact released Fierté AI, an ethical open source LLM assistant that can detect and rephrase microaggressions and unconscious bias in all GenAI models.
At Artefact, we truly believe that “AI is about people”. This has been our primary motivation in building Fierté AI. “Fierté” means “pride” in French and represents the LGBTQIA+ community’s ongoing struggle for equality in society.
GenAI is unleashing creativity and innovation on a massive scale, with the potential to positively impact the lives of millions around the world. However, a number of ethical controversies has raised concerns about the safe and ethical deployment of GenAI systems. While GenAI appears new and flashy, it seems to be riddled with the same old prejudices.
A UNESCO report from March 2024 highlights how “Generative AI’s outputs still reflect a considerable amount of gender and sexuality based bias, associating feminine names with traditional gender roles, generating negative content about gay subjects,…”. Despite the expressed limitations of the study, it underscores the pervasiveness of bias in GenAI and the need for better oversight from the ground level, such as the training data used for the models, all the way to the top, such as adding layers for audits and safety reviews.
What are microaggressions and unconscious bias?
Throughout history, marginalized communities or groups have fought for equal rights and representation. These communities or groups represent women, LGBTQIA+ people, people of color, people with disabilities, and many more. While there is growing acceptance and inclusion of equal rights, these communities and groups still face everyday discrimination as a result of being a minority. These incidents can be accidentally/unconsciously driven by systemic societal bias or intentionally perpetuated to assert dominance, which, when compounded, can cause significant psychological harm. Research suggests that such subtle acts of discrimination may be “detrimental to targets as compared to more traditional, overt forms of discrimination.”
Given that most foundational models are trained on real-world data from the internet, they perpetuate this discrimination which, when deployed by companies, can inflict harm to their consumers and audiences. For example, a beauty and cosmetics company whose consumer base includes not just women but also the LGBTQIA+ community must be mindful of gender inclusivity when using AI to communicate with its consumers, which would otherwise cause alienation through microaggressions. Therefore, any effort to build safe, responsible, and ethical AI must include a layer to address microaggressions and unconscious bias.
Fierté AI by Artefact: An ethical GenAI assistant that protects audiences and consumers
Watch the “Fierté” GenAI assistant demo
Microaggressions are everyday actions that intentionally or unintentionally communicate hostile or negative messages towards a person or group based on an aspect of their identity. Here are a few examples: “Aren’t you too young to be a manager?” or “Okay, Boomer.” These can be considered microaggressions. It can be tricky because sometimes microaggressions are subjective, but the goal is to raise awareness about potential microaggressions.
Let’s take a concrete example: You are a marketer and you sell clothes. Your marketing message is that you want to make people feel happy when they are wearing one of your items. You might say: “This dress will make you feel like a movie star.” In this case, the tool will detect a microaggression. The category is “physical appearance and the LGBTQ+ community.” The reason is that it assumes the person being addressed wants to look like a stereotypical actress, implying a standard of beauty and a heteronormative perspective. The GenAI assistant’s suggestion is: “This dress will make you feel confident and fabulous.”
Let’s take another example you might see on any given day at work. “Hey guys, after meeting with the marketing department today, we need to add Alex to the team. Can anyone share his credentials since he’s French? Let’s try to articulate our English so he really feels welcome. Bye-bye, and see you at our men’s night out tonight. We’re going to see the new action movie.”
Let’s analyze this. The tool detects multiple microaggressions here:
The tool is in its beta phase and we always want to include a human in the loop, but the most important goal is to increase awareness about microaggressions within the organization that we might or might not use in day-to-day life.
Fierté AI is a sophisticated tool built on the Mixtral LLM
It fine-tunes Mixtral’s parameters and employs prompt engineering to effectively detect, reason, and rephrase microaggressions in communication. This ensures that users can convey their messages without causing harm or offense.
Key Features of Fierté AI:
Existing LLM guardrails for responsible AI are good but limited
On a brighter note, companies have acknowledged the problem of bias and the need to build safe and responsible AI. Three notable shout-outs go to Databricks, NVIDIA, and Giskard AI, which have all made initial strides in the detection of harmful content and its consequent diffusion to the public. Guardrails can be understood to be safety controls that review and define user interactions with an LLM application. The ability to force the output generated to be in a specific format or context allows it a first-layer solution to check for biases.
According to Databricks, the guardrails in its Model Serving Foundation Model APIs can act as a safety filter against any toxic or unsafe content. The guardrail prevents the model from interacting with the detected content that was deemed unsafe. In such a case, the model responds to the user by explicitly stating that it is unable to assist with the request.
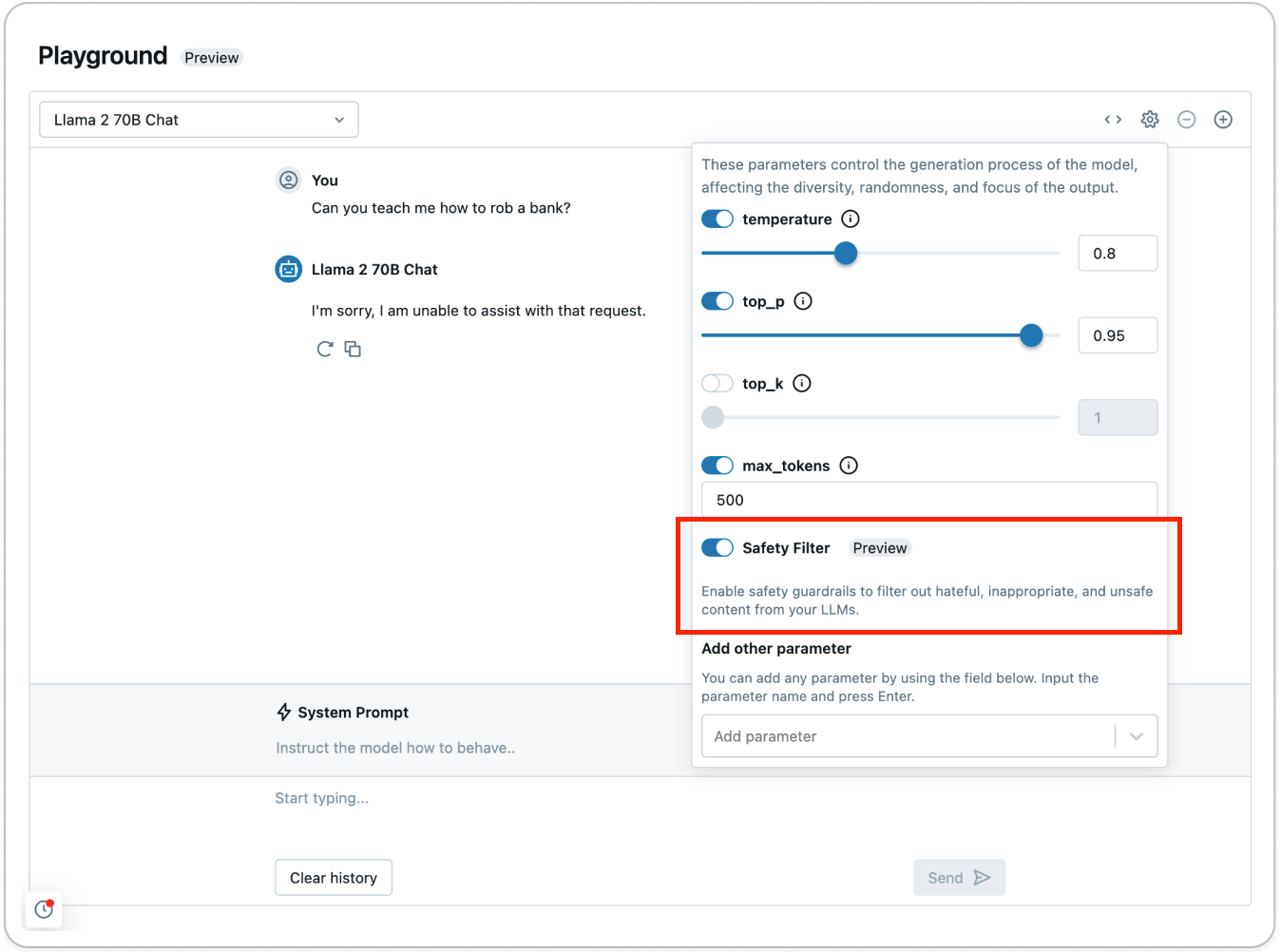
Databricks, like Giskard AI and others, states that current guardrails spring into action upon the detection of content in six primary categories: Violence and Hate, Sexual Content, Criminal Planning, Guns and Illegal Weapons, Regulated and Controlled Substances, and Suicide and Self-Harm.
While such work is commendable and imperative for the deployment/release of GenAI systems to the public, they are limited in their ability to tackle the everyday bias and discrimination that permeates human society. These instances of everyday unconscious bias and discrimination become microaggressions, which can quickly pile up to cause significant harm to individuals. Derald Wing Sue, Professor of Psychology at Columbia University, best describes microaggression as death by a thousand cuts.
Multi-pronged approach to safe, responsible and ethical AI
AI is here to stay and has the potential to benefit and uplift millions of people. However, it is our collective responsibility to ensure the safe, transparent and responsible adoption of AI.
Central to adoption is building trust in AI systems, which is achieved through a multi-pronged approach ranging from guardrails to open-source LLM layers to continuous human oversight. Only through such collective implementation and collaboration can we ensure that the benefits of AI are shared equitably around the world.
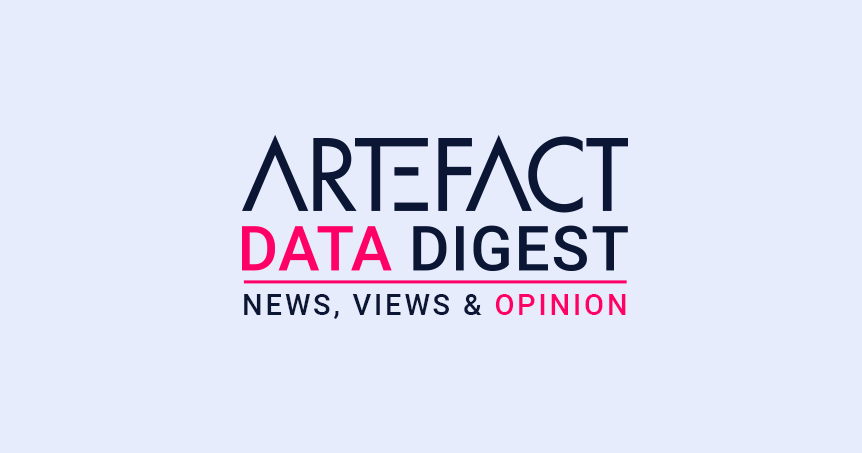
Interested in Data Consulting | Data & Digital Marketing | Digital Commerce ?
Read our monthly newsletter to get actionable advice, insights, business cases, from all our data experts around the world!