From Studio to Streaming: AI Influence in Shaping Music and Its Market Impact
The first documented live performance blending generative AI and a musician took place in November 2022, when pianist David Dolan (Guildhall School of Music and Drama) improvised in dialogue with a semi-autonomous AI system designed by composer Oded Ben-Tal (Kingston University). This groundbreaking concert demonstrated the creative possibilities of AI as a collaborative partner in music. Since then, musicians and even non-musicians have embraced generative AI, producing full compositions with vocals, instruments, and even sounds from non-existent instruments. This marks the beginning of a new era where AI is reshaping music creation and production. As artificial intelligence continues to reshape industries, the music world is experiencing its own revolution. From hobbyists to major record labels, stakeholders across the industry are grappling with the implications of this technology.
The music industry, historically shaped by technological advancements, is entering a new phase of transformation. With the music industry’s broad impact across entertainment, media, and streaming platforms hosting millions of tracks, the integration of AI promises to redefine how music is created, produced, and consumed.
Generative AI, the technology behind this seismic shift, has rapidly evolved from academic research to practical applications. Models like Music Transformer and MusicLM are pushing the boundaries of what’s possible, translating abstract concepts into harmonious compositions. These AI systems, trained on vast datasets of musical pieces, can now generate original melodies, harmonies, and even entire songs with astonishing proficiency.
However, as with any technological revolution, the rise of AI in music brings both excitement and anxiety. Artists and industry professionals are divided on its potential impact. Some see it as a powerful tool for creativity and democratization, while others worry about the implications for human artistry and job security.
The landscape of the music industry is already changing. Streaming platforms are leveraging AI for personalized playlists, while production software is incorporating AI-assisted mixing and mastering tools. Even major record labels are exploring AI’s potential for talent scouting and trend prediction.
As we delve into this new era, questions abound. How will AI reshape the roles of artists, producers, and engineers? What legal and ethical considerations must we address? And perhaps most importantly, how will this technology affect the emotional connection between musicians and their audience?
In this article, we’ll explore the current state of generative AI in music, its applications across different sectors of the industry, and the potential future it heralds. From the technology behind these AI models to the practical challenges of bringing them to market, we’ll examine how this innovation is poised to transform one of the world’s most beloved and influential creative industries.
As the music industry enters this AI-driven era, it’s evident that future composers may not be solely human, marking a significant shift as the industry navigates this new frontier.
1. The Technology Behind Generative AI for Music
From Composition to Code: The Breakthroughs of Generative AI in Music
The journey from traditional composition to AI-generated music has been nothing short of ground breaking. We are familiar with text generation using chatGPT and image generation with Stable diffusion and MidJourney. Music generation major breakthroughs lie in the adaptation of those powerful models to the specificities of music data.
I. GPT for Music (discrete generation)
Just as GPT models process language, similar principles can be applied to musical elements. The key lies in tokenization—breaking down music into discrete, manageable units. The process of applying GPT models to music through tokenization is a crucial step in enabling AI to understand and generate music. Here’s a more detailed explanation:
1. Tokenization in music: Just as GPT models break down text into tokens (words or subwords), music needs to be broken down into discrete units that the AI can process. We explore below two tokenization methods:

i. Notes can be seen as discrete element containing multiple informations :
a) Pitch: The specific note being played (e.g., C, D, E, etc.)
b) Duration: How long the note is held (e.g., quarter note, half note)
c) Velocity: How loudly or softly the note is played
d) Instrument: Which instrument is playing the note
ii. Example : a C4 note played for a quarter beat might be represented with tokens : ; ; , while a G3 note played for a half beat might be tokenized into ; ;
( we can add tokens for velocities, bar structures, instruments, genres,…)
iii. Numerical Sequence creation:
These series of notes-tokens are then concatenated and arranged in sequences, similar to how words form sentences in language models. Each token is assigned a unique numerical ID, enabling the GPT model to receive a similar input as for text generation.
iv. Example : A musical phrase looks like: [123; 8; 456; 118; 12; 451;…] where each number represents a specific musical event.
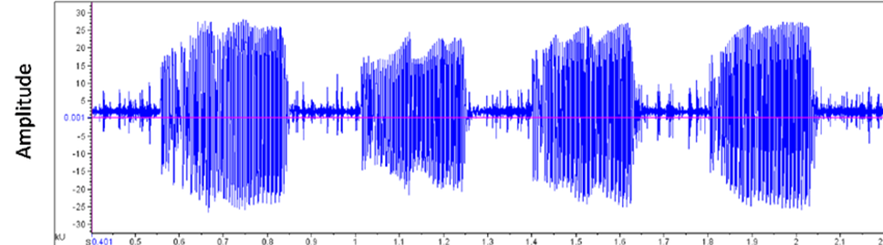
i. Audio files are stored in computers in a wave format, representing the amplitude of the signal over time. Which provides a richer representation than simple music sheets.
ii. This wave can be divided into segments representing the “meaning of music” using a trained model (similar to BERT for word vectors) which creates a tokenized version of the audio input.
iii. The resulting vectors can be conditioned by a joint audio and text embedding, creating tokens representing both audio characteristics and textual description, enabling the user to use a text-prompt as input during inference.
2. AI training:
3. Generation:
Music Transformer, developed by Google’s Magenta project, marked a significant milestone in this field. By being among the first to apply the Transformer’s Attention mechanism—a cornerstone of language models—to musical sequences, it achieved remarkable coherence in long-form compositions. Other models followed suit, each pushing the boundaries of what AI could accomplish in music creation.
II. Diffusion models for music (Continuous generation)
Diffusion models, such as MidJourney or Stable Diffusion, have been largely adopted and used for image generation. The generated images are getting more and more detailed and customizable showing great consistency while adding style or resolution details in a prompt. This personalization is an interesting feature for music generation as well. How can we turn music into an image?
Music as a picture:
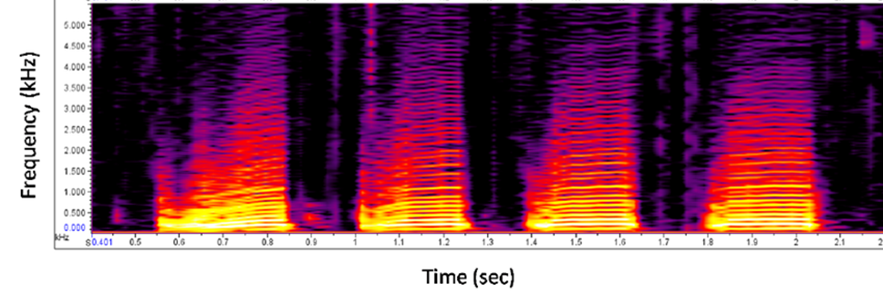
By considering spectrograms as pictures and aligning them with a text description, it is possible to have a powerful control over the generation, enabling users to prompt their desired music as they would do for pictures with MidJourney.
Bridging the Gap from Innovation to Industry: Challenges in AI Music Generation:
We have explored 2 different ways to generate music using AI. All of these technologies led to impressive and entertaining demos. However, the path from laboratory experiments to market-ready products is fraught with challenges. Technical hurdles abound, from ensuring consistent quality across various musical styles to managing the computational demands of real-time generation. Market challenges are equally daunting, as developers grapple with finding the right audience and use cases for their AI composers.
Management and legal considerations add further complexity. Building and leading teams at the intersection of music and AI requires a unique blend of skills. Meanwhile, the ethical and legal landscape remains largely uncharted, raising questions about copyright, authorship, and the very nature of creativity in the age of AI.
As we delve deeper into the current applications and products emerging from this technological revolution, it becomes clear that the impact of AI on the music industry will be far-reaching, touching every stakeholder from listeners to major record labels.
2. Current Applications and Products
As generative AI continues to develop, a variety of platforms and tools have emerged in the music industry. These innovations are moving from experimental concepts to practical applications, each offering different capabilities and potential uses.
I. The most used today: Suno.AI
In the landscape of AI music generation, Suno.AI has gained significant attention. The platform allows users to create music from text prompts, generating full songs including vocals, instrumentation, and lyrics in various genres and styles.
Suno.AI has reported a user base of 12 million and completed successful funding rounds, reaching a valuation of $500 million. The platform offers both free and paid tiers, with the free version allowing users to create a limited number of songs per day. Its applications range from film trailer mockups to video game soundtracks.
A filmmaker working on a short film might use Suno.AI to generate a custom soundtrack when unable to hire a composer. Advertising agencies are exploring the platform to quickly create jingles and background music for commercials, potentially altering their workflow for music in advertising.
II. Other AI Music Platforms
While Suno.AI has garnered attention, it’s part of a growing ecosystem of AI music platforms.
As these platforms evolve and new ones emerge, the applications of AI in music continue to diversify. From individual creators to major corporations, the potential uses span a wide range, indicating a future where AI could play an increasingly significant role in music creation and production.
3. Impact on Different Stakeholders
As generative AI continues to evolve, its impact reverberates through every facet of the music industry. From listeners to major record labels, each group is experiencing a shift in their relationship with music creation and consumption. While some view these changes with anxieties, others see them as the natural progression of an industry that has always been shaped by technological advancements.
I. Listeners
The journey for music consumers has been one of increasing personalization and discovery. Before AI, listeners relied on radio DJs, music magazines, and word-of-mouth to discover new music. Playlists were painstakingly curated by hand, often limited by personal music libraries.
With the advent of AI, platforms like Spotify introduced features such as Discover Weekly in July 2015, using algorithms to analyze listening habits and suggest new tracks. This marked a significant leap in personalized music discovery, exposing listeners to a broader range of artists and genres.
Now, with generative AI, we’re on the verge of an even more tailored experience. LifeScore is a system that not only recommends existing music but creates new compositions based on a listener’s mood, activity, or even biometric data. A jogger might have a custom soundtrack generated in real-time, adapting to their pace and heart rate, blending their favorite genres into something entirely new.
II. Artists
For musicians, the creative process has always been influenced by available tools and technologies. Before AI, composition relied heavily on traditional instruments and recording techniques. The introduction of synthesizers and digital audio workstations (DAWs) in the late 20th century already began to reshape how music was created. As noted by Jean-Michel Jarre, an early electronic music pioneer :”Technology has always dictated styles and not the other way around. It’s because we invented the violin that Vivaldi made the music he made… For me, AI is not necessarily a danger if it is used well”.
Composition software can suggest chord progressions or melodies, serving as a collaborative partner in the creative process.
With generative AI, we’re seeing the emergence of AI co-writing. Artists can now input a basic melody or lyrical idea and have an AI system generate complementary elements, potentially sparking new creative directions. Some musicians are even experimenting with real-time style adaptation during live performances, using AI to morph their sound on the fly based on audience reactions.
For amateur artists, multiple training apps are making good use of AI to propose real-time, interactive and personalized learning experiences. With other tools enabling you to simulate arrangements and bands, the learning cost of creativity is reduced, enabling more people to play and enjoy music.
III. Engineers
The role of audio engineers has been one of constant adaptation to new technologies. Traditionally, mixing and mastering were entirely manual processes, requiring a keen ear and years of experience. Interrogated for this article, Marius Blanchard, Data Scientist at Spotify’s mentioned: “AI is not here to replace musicians, but to empower them. It’s a tool that can help artists explore new creative territories and push the boundaries of music creation.”
AI has already made inroads in this domain, with tools like iZotope’s Neutron offering intelligent mixing assistance. These systems can analyze a track and suggest EQ settings, compression levels, and other parameters, streamlining the mixing process.
As generative AI becomes more sophisticated, we may see fully automated mixing and mastering systems that can process an entire album with minimal human intervention. However, many in the industry argue that the human touch will always be necessary to capture the emotional nuances of a performance.
IV. Music & Platform Companies
Record labels and music publishers have long relied on human intuition and market research to spot trends and discover new talent. A&R (Artists and Repertoire) executives would scour clubs and demo tapes, looking for the next big thing.
The integration of AI has shifted this process towards data-driven decision making. Platforms analyze streaming data, social media engagement, and other metrics to identify rising artists and predict hit potential.
With generative AI, music companies are exploring even more radical possibilities. Some are experimenting with AI systems that can generate music tailored to specific market segments or demographics. Others are using predictive models to forecast which song structures or lyrical themes are likely to resonate with audiences in the coming months.
As the industry grapples with these changes, the key question remains: How will the balance between human creativity and AI assistance evolve? While some fear the loss of human touch in music creation, others see AI as a tool that will ultimately enhance human creativity, opening new avenues for expression and collaboration.
4. Future Visions and Opportunities
As generative AI continues to reshape the music industry, stakeholders are grappling with a complex landscape of legal, economic, and ethical considerations. This transformative technology promises to democratize music creation while simultaneously challenging traditional notions of artistry and copyright.
I. Legal & Market Considerations
1. Copyright in the Age of AI
Before AI’s emergence, copyright laws in music were relatively straightforward. Composers and performers held rights to their original works, with clear guidelines on sampling and fair use. However, the introduction of AI-generated music has blurred these lines significantly.
In the U.S., copyright protection extends to original works of authorship, while European doctrine emphasizes the creator’s personal touch. This distinction becomes crucial when considering AI-generated music. The U.S. Copyright Office has stated that it will not register works produced by a machine or mere mechanical process that operates randomly or automatically without creative input or intervention from a human author.
In 2023, at least one high-profile case highlighted copyright concerns around AI-generated music mimicking popular artists. A track called “Heart on My Sleeve” that used AI to imitate the voices of Drake and The Weeknd was uploaded to streaming services and then promptly removed. Universal Music filed a claim on YouTube, and the track’s video was taken down. This incident raised issues around personality rights and the unauthorized use of artists’ voices, even if created through AI This action set a precedent for how the industry might handle AI-created content that closely resembles existing artists’ work.
For artists, AI presents a double-edged sword. Some embrace the technology as a collaborative tool, while others fear it may devalue their craft.
Music companies face their own set of challenges.
2. Market Projections
AI in the music market is poised for significant growth. Industry analysts project the sector to reach $38.7 billion by 2033, up from $3.9 billion in 2023.This tenfold increase reflects the anticipated widespread adoption of AI tools across various aspects of music production, distribution, and consumption. Interestingly, artists are more looking for AI powered tools in music production and music mastering (66%) rather than for AI music generation (47%). Creativity-enabling tools are seen as more important than pure AI-generation tools, even if we have to prepare ourselves to listen to more and more AI-generated music extracts.
II. Potential Transformations
1. Democratization of Music Production
AI is lowering the barriers to entry for music creation. Tools that once required expensive studios and years of training are now accessible through user-friendly apps. This democratization could lead to an explosion of new musical styles and voices.
A music tech startup founder, Alex Mitchell, CEO of Boomy, envisions a future where anyone can be a composer. “Now, we’re seeing people all over the world create instant songs with Boomy, release them, and even earn royalty share income. For the first time, musical expression is available to an entirely new type of creator and audience” . This accessibility could fundamentally alter the music landscape, potentially unearthing talent that might otherwise have gone undiscovered.
2. New Artist-Audience Interactions
AI is indeed reshaping how artists connect with their audience. Some artists and music platforms are already experimenting with AI-powered chatbots that allow fans to interact with their music catalog in novel ways. For example, Spotify introduced an AI-driven chatbot within its application that interacts with users to understand their music preferences, moods, and listening habits. This allows the chatbot to curate customized playlists and suggest new tracks tailored to each user’s unique taste.
These AI chatbots are being used to enhance fan engagement by providing personalized recommendations, real-time updates, and interactive experiences. They can offer artist updates, recommend playlists, facilitate ticket purchases, and manage fan inquiries, fostering deeper connections between artists and their audience.
A forward-thinking artist, Holly Herndon, recently launched an AI version of her voice that fans can collaborate with to create personalized tracks. This blending of human creativity and AI capabilities points to a future where the line between artist and audience becomes increasingly fluid.
A Multifaceted Conclusion
I. AI’s Role in Creativity
The integration of AI in music creation raises profound questions about the nature of artistry. While some argue that AI is merely a tool, akin to a new instrument, others fear it may diminish the human element that makes music emotionally resonant.
Marius Blanchard, Data Scientist from Columbia University and working in the music industry offers a balanced perspective: “I do not believe AI will ever replace artists; […] the audience-artist connection lives on many different levels beyond the music. Instead, I hope that AI will democratize access to quality sound production and be a creativity boost for more and more artists to express and share their creations.”
II. Impact on Industry Jobs
As AI capabilities grow, certain roles within the music industry may evolve or become obsolete. Sound engineers, for instance, may need to adapt their skills to work alongside AI mixing and mastering tools. However, new job categories are likely to emerge, such as AI music programmers or ethical AI consultants for the creative industries.
III. Authenticity and Emotional Connection
Perhaps the most significant question facing the industry is whether AI-generated music can forge the same emotional connections with listeners as human-created works. While AI can analyze patterns and create technically proficient compositions, the ineffable quality of human experience that often infuses great music remains a challenge to replicate.
Electronic musician and AI researcher Holly Herndon offers an optimistic view: “I think the best way forward is for artists to lean into developments with machine learning,” she said, suggesting that they “think of ways to conditionally invite others to experiment with them.”
References
Jeff Ens and Philippe Pasquier, (2020). MMM : Exploring Conditional Multi-Track
Music Generation with the Transformer. arXiv preprint arXiv:2008.06048 https://arxiv.org/abs/2008.06048
Andrea Agostinelli et al, (2023). MusicLM: Generating Music From Text. arXiv preprint arXiv:2301.11325 https://arxiv.org/abs/2301.11325
Huang et al, (2018). Music Transformer: Generating Music with Long-Term Structure. arXiv preprint arXiv:1809.04281 https://arxiv.org/abs/1809.04281
Ke Chen et al, (2023). MusicLDM: Enhancing Novelty in Text-to-Music Generation Using Beat-Synchronous Mixup Strategies. arXiv preprint arXiv:2308.01546 https://arxiv.org/abs/1809.04281