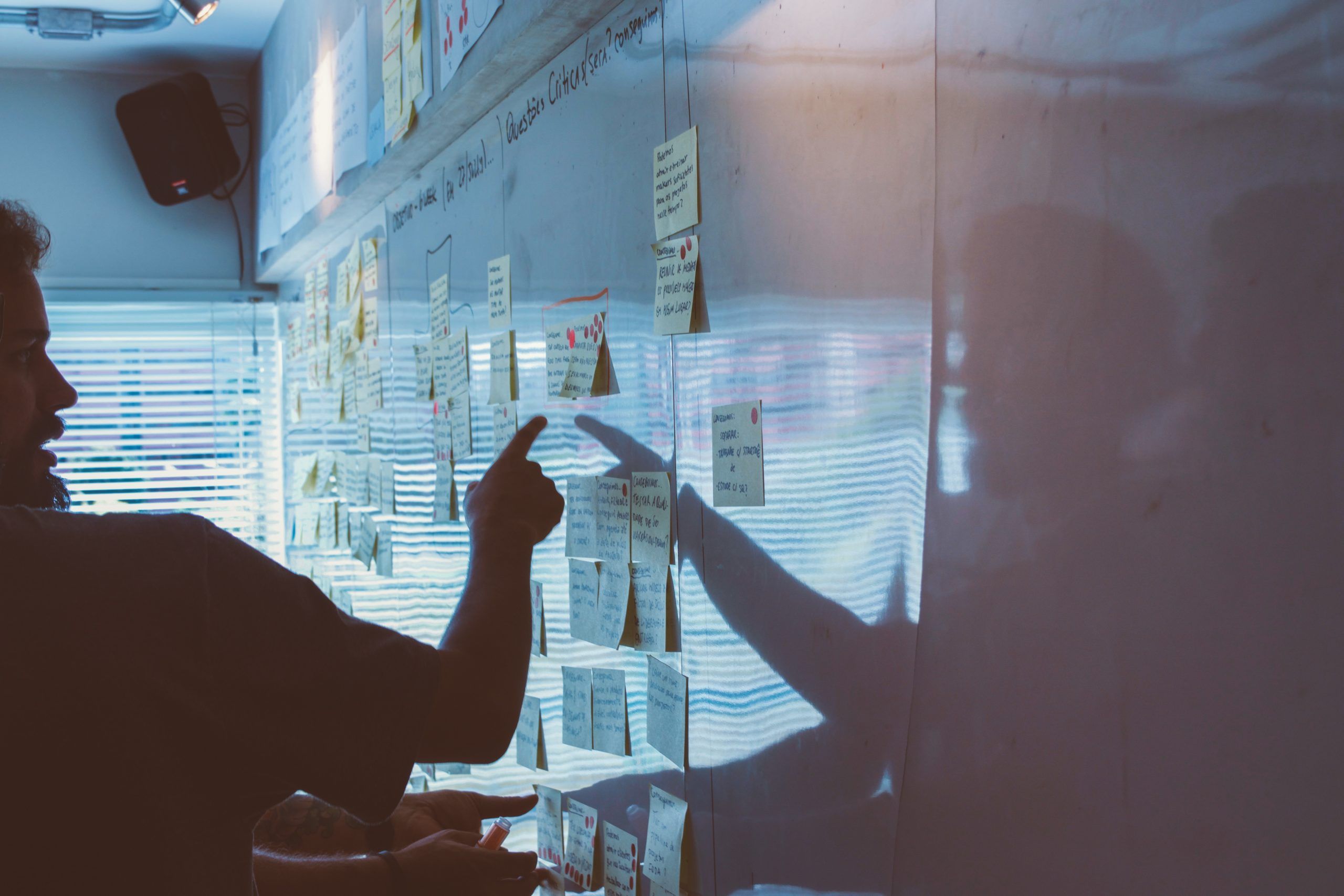
NEWS / AI TECHNOLOGY
The increasing understanding of and experience with artificial intelligence by organisations might seem contradictory given the lack of significant AI implementation within most enterprises.
The increasing understanding of and experience with artificial intelligence by organisations might seem contradictory given the lack of significant AI implementation within most enterprises.
In this article, we will examine both the underlying reasons for this dichotomy and explore the conditions required to achieve success.
Organisations which are successfully implementing AI technologies are seeing the emergence of new models, especially in the form of an “AI factory”, a combination of talents, methods, and technologies at the service of the entire company.
Innovation in nascent technologies invariably follows Gartner’s branded “Hype Cycle”, which identifies five overlapping life cycle stages:
- Innovation Trigger: In this stage, a potential technology gets things started. There may be prototypes, and lots of media interest and publicity, but frequently there are no commercially viable products available.
- Peak of Inflated Expectations: At this point, the technology is implemented; it produces a number of success stories – but far more failures. Some companies adopt the technology; many do not.
- Trough of Disillusionment: Interest begins to fade as flaws and failures come to light. Some producers of the technology drop out of the race. Investments continue only if the surviving providers improve their products to the satisfaction of users.
- Slope of Enlightenment: Ways in which the technology can benefit companies become more apparent. More enterprises test it; some companies produce second and third generation products.
- Plateau of Productivity: The technology becomes widely implemented. Its market applications become clear and high-growth adoption starts.
As the cycle demonstrates, each phase of concrete success is preceded by a phase of disillusionment. Too many complications can impede the success of these projects. The stakes of meeting the challenge of Artificial Intelligence are high: according to Accenture, enterprises which make good use of artificial intelligence could see their profitability grow by more than 30%! Added to this are potential new sources of revenue, which may result from enhanced customer experiences or increased competitive advantage.
What is AI? What value can it bring to businesses?
AI, which is still in its infancy, is an ensemble of highly theoretical sciences; nevertheless, it must be viewed by enterprises with a view to cost savings, and not through the prism of innovation (the “latest buzzword” syndrome). How can companies draw real value from these AI experiments? How can they succeed in “scaling up”? Our answer demands new organizational frameworks where we speak of “AI factories”.
Today, more and more algorithms and “intelligence products” are on the shelves. At least on the lower levels. Europe’s first Cloud partners, Amazon (AWS), Microsoft (Azure), Google (GCP), and IBM, all offer various levels of abstraction which enable companies to “produce” AI in-house (Google recently launched a new managed universal service called Anthos); these solutions work a bit like the chemistry kits we played with as children. They propose simple access to infrastructures specifically designed to respond to machine learning needs, such as Google’s TPUs, which are managed services enabling the concentration of teams on given applications.
Also available are numerous open source libraries (scikit-learn, TensorFlow, Pytorch) as well as proprietary APIs (image, text, and voice recognition). But problems may arise after initial successes, as only a small part of these tests end up in production mode, generating not only frustration but, obviously, lost opportunities.
Most large organisations have massively invested in Data Science, and rightly so: in 2016, McKinsey announced that 250,000 Data Scientists would be needed by 2021 in the US alone. But we’re now realizing that these profiles, which are highly focused on data models, statistics, and algorithms, will not be sufficient to transform the enterprise with AI. We omitted, for example, the invaluable Data Engineers and Data Architects whose talents are key to ensuring success in the coming years, but whose integration into team projects has yet to be achieved.
A new organizational mode is gradually coming to light: The Artificial Intelligence factory, or AI factory,
which follows a certain number of guiding principles which are simple but crucial to the success of these initiatives.
The AI Factory begins with centralized governance
One with very ambitious goals… The idea is to pool and coordinate investment and steering efforts. Only a very small number of the company’s highest-value projects will be examined by those sponsors most engaged in their success. The selection of these use cases must be extremely rigorous: specifically, no project should see the light if it doesn’t respect the simple law of 10X (offer a 10:1 return on investment). The success and impact of each use case should be measurable according to a simple and understandable KPI, and the systematic improvement of this KPI should be the raison d’être of the teams.
Feature teams ensure dedicated project organisation
Popularized by Spotify, feature teams respond to the challenges of reducing time to market, transversality, and project continuity. Directed by a business manager, the feature team is composed of a product owner, data scientists, data engineers and DevOps experts. The inclusion of DevOps / IT in the feature team ensures excellent supervision and perennial maintenance of the AI solution. A “platform team” ensures the technological coherence of the building blocks deployed by the feature teams. It is important to note that this organizational model works very well at scale (there are up to 10 feature teams for each “Tribe” at Spotify.)
The Benefits of Feature Teams
- Feature teams are better able to evaluate the impact of design decisions. At the end of a sprint, a feature team will have built end-to-end functionality, traversing all levels of the technology stack of the application. This maximizes members’ learning about the product design decisions they made (Do users like the functionality as developed?) and about technical design decisions (How well did this implementation approach work for us?)
- Feature teams reduce waste created by hand-offs. Handing work from one group or individual to another is wasteful. In the case of a component team, there is the risk that too much or too little functionality will have been developed, that the wrong functionality has been developed, that some of the functionality is no longer needed, and so on.
- It ensures that the right people are talking. Because a feature team includes all skills needed to go from idea to running, tested feature, it ensures that the individuals with those skills communicate at least daily.
- It keeps the focus on delivering features. It can be tempting for a team to fall back into its pre-Scrum habits. Organizing teams around the delivery of features, rather than around architectural elements or technologies, serves as a constant reminder of Scrum’s focus on delivering features in each sprint.
A specific methodology: Lean AI
Lean AI is a methodology which reduces the uncertainty of efficiency and applicability of AI solutions. Models are never perfect and must be tested in real-world situations. The method consists of a continuous improvement loop of short cycles which include the formulation of hypotheses, the identification of pertinent data, the construction and testing of one or more models, followed by deployment on a test perimeter and collection of user feedback.
The cycle is repeated with the formulation of new hypotheses, new data, etc. This method enables testing in real situations, then the improvement of cases not explored, until reaching a level of satisfaction considered acceptable by the organisation in order to begin production.
… Along with an adapted infrastructure
Deployment should be envisioned from the very first days of the project in order to avoid starting from scratch in a different technical environment.
The creation of new data silos must also be avoided by making maximum use of the organisation’s existing data lakes and data wells. AI applications should be built in a service-oriented architecture logic.
Containerisation and orchestration technologies such as Docker and Kubernetes enable simplified management of microservice ecosystems, facilitating the use of AI models via APIs which can be used by all the organisation’s divisions.
… And work on internal transformation and adoption
Although many companies already share a common space, the lack of sharing a common operational model is undermining the success of AI projects. For example, a “Chatbot” belonging to a commercial website should be a natural part of the sales path, rather than be considered as a separate acquisition silo, yet this is often not the case. When the different departments across an enterprise adopt the same technologies, AI success is far more likely.
Steps to AI project success
#1 Define your AI Goal
2 Back it with Data
#3 Involve your in-house Subject Matter Experts (SMEs).
#4 Measure outcome, not output
#5 Iterate Fast!
Finally: an ever more important ethical challenge
We have recently seen the example of Alexa and the unpleasant surprise of her listening (necessary and planned in order to improve supervised learning). Regulations will always lag behind technology. It is important that those enterprises which employ artificial intelligence understand the ethical challenges of these solutions. Seven guiding ethical principles, which were published by the Committee of Independent Experts mandated by the European Commission: AI at the service of humanity, trustworthy, which respects private data, transparent, non-discriminatory, dedicated to the improvement of the common good, and finally, with a clearly defined human responsibility.
A growing number of organisations are deciding to apply these principles to extract the value of AI: Carrefour recently announced the launch of their Artificial Intelligence Laboratory in partnership with Google, and Walmart announced the launch of its “Cloud Factory” in Austin with Microsoft. There is little doubt that these new organisational methods, focused on value and pragmatic in their approach to innovation, will be more frequently and successfully deployed in the coming years.