Introduction
Many theoretical articles have been written about data governance. At Artefact, we want to address this topic from an operational standpoint with our article series, “Insights from the field”, to give our readers pragmatic and actionable insights. The series will be composed of Artefact’s observations and feedback on key data governance topics (e.g. operating models), answers to questions frequently asked by our clients and prospects (e.g. how to measure the impact of data governance), and interviews with key actors in data governance (e.g. Chief Data Officers, Chief Governance Officers, data custodians, software editors, etc.). Our ambition is to create conversations and opportunities to share experiences within the data governance community.
Understanding what data governance is, why it is on the critical path to value and how to concretely deploy it in your organisation can be a long road. When companies first launched large transformation programmes with AI and data use cases, they quickly realised these programmes required key enablers such as data platforms, data lakes, data quality management and MDM, which allow quality data to be exposed to use cases. Only recently has data governance appeared as a priority with regard to the myriad of complex systems created.
Understanding what data governance is, why it is on the critical path to value and how to concretely deploy it in your organisation can be a long road. When companies first launched large transformation programmes with AI and data use cases, they quickly realised these programmes required key enablers such as data platforms, data lakes, data quality management and MDM, which allow quality data to be exposed to use cases. Only recently has data governance appeared as a priority with regard to the myriad of complex systems created.
Today, almost everyone in the CDO/CTO ecosystem understands that data governance is a prerequisite to AI transformation. They’re familiar with all the basic organisational and operational concepts, but setting them to music to deliver concrete value is a far more complex task. As a result, data governance is often reduced to isolated documentation initiatives with little impact. We see many programmes being launched, and many heads of data governance appearing in our clients’ organisations. At first, they all encountered the same difficulties in convincing their sponsors and business partners to invest in these activities. Why? Because deploying data governance is a complete learning process that the organisation has to go through, and each step along the path is important.
The purpose of this report is to help you understand what data governance is in practice by taking you on a journey through the data governance learning process each company inevitably experiences. This new awareness will allow companies to move more quickly from one stage to the next.
We will achieve this by explaining each stage of the learning process in detail, using operational examples from the field:
- Stage 1 – Unconscious incompetence: what are the “symptoms” of a lack of data governance?
- Stage 2 – Conscious incompetence: what are the challenges encountered by a company when starting a data governance journey?
- Stage 3 – Conscious competence: what are the steps companies usually follow when launching a data governance programme?
- Stage 4 – Unconscious competence: what happens when data governance becomes the new normal?
This article will be followed by more operational ones designed to offer pragmatic advice to data governance leaders in their journey.
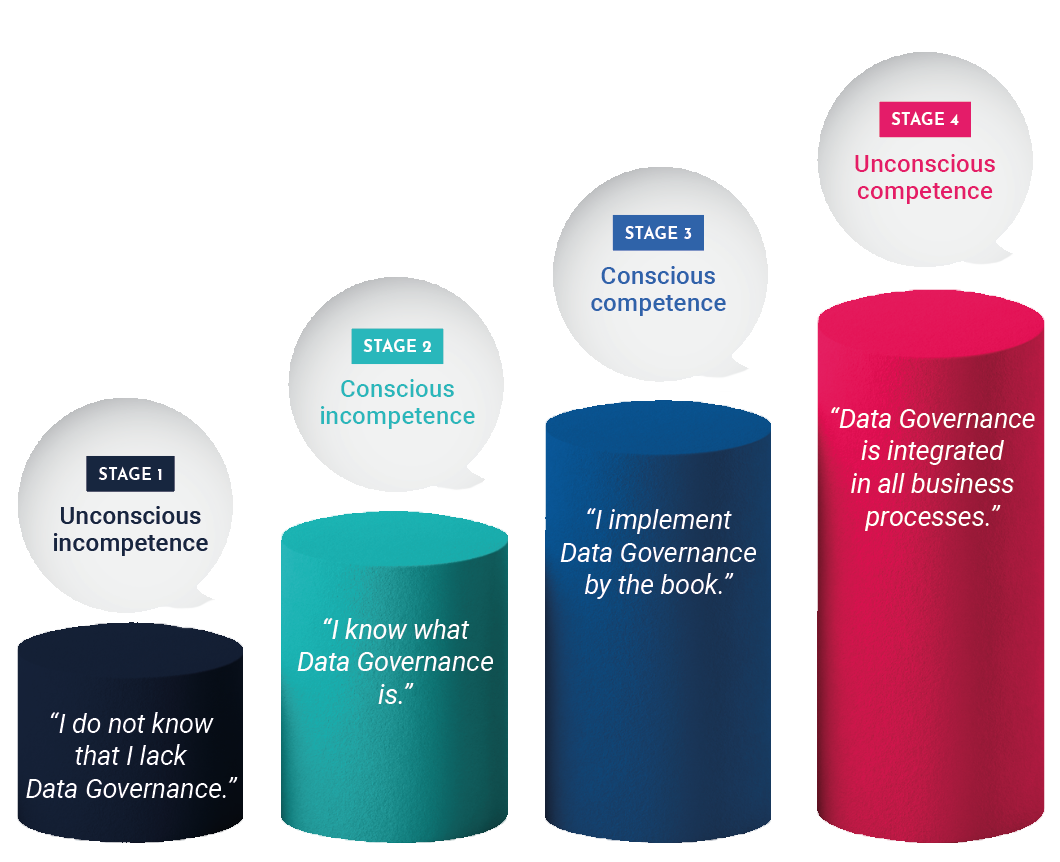
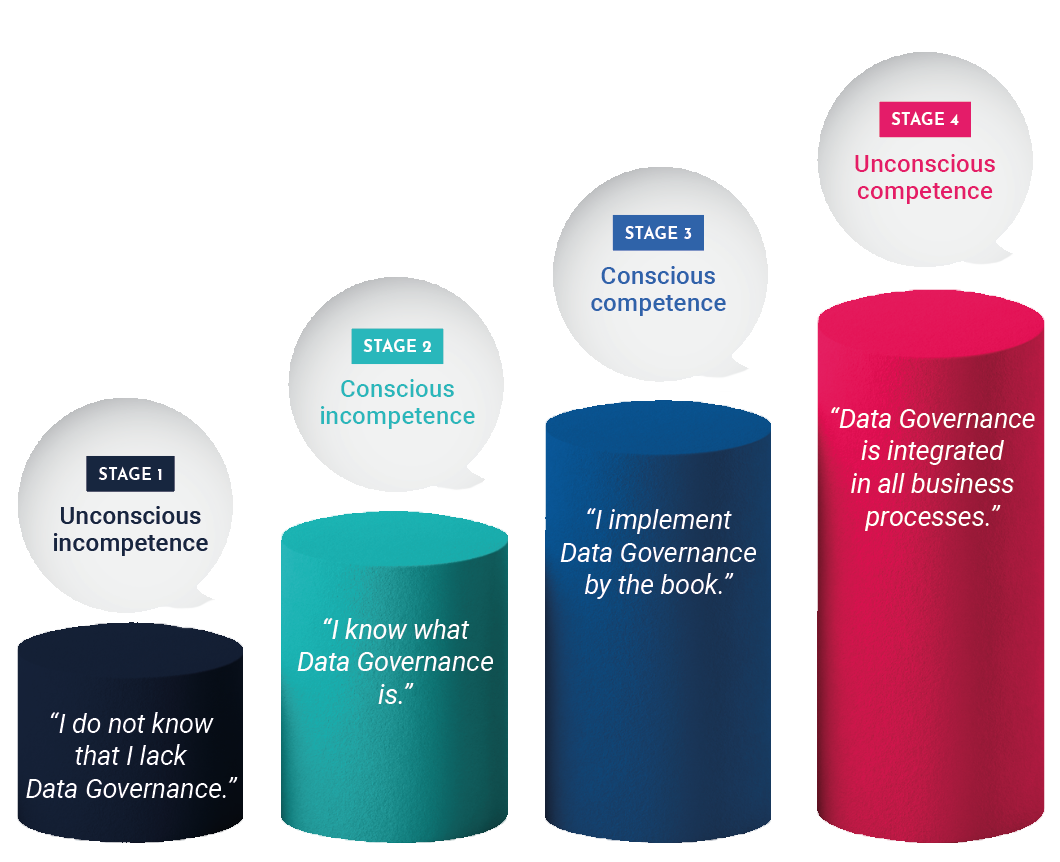
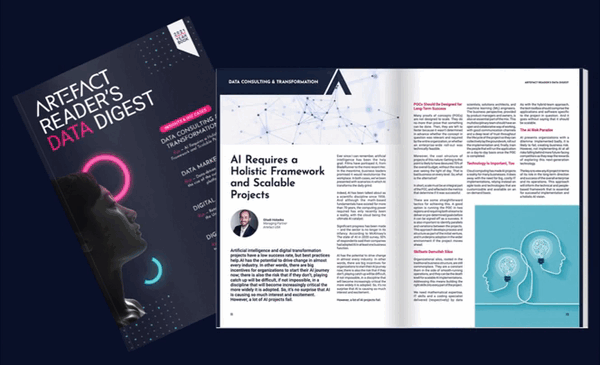
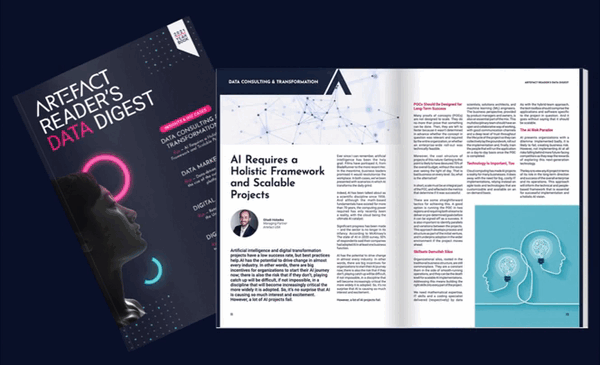
Conclusion
By following these four learning stages, companies will begin practising data governance without even realising it.
Although the majority of actors we’ve accompanied today still hover between the second and third stages, they all feel the business urgency to move forward in this learning process.
We see three main arguments that justify this urgency. First, data transformation is a key lever in all industries to achieve ambitious goals: data and AI are leveraged to optimise companies’ core businesses and contribute to the development of new growth drivers. Becoming data and AI mature is a requirement in order to remain on the cutting edge, and data transformation isn’t sustainable without data governance. The second argument is that we’re in a fast-moving world. Obtaining speedy access to data and developing the capacity to rapidly analyse and exploit this data is essential to react quickly. And finally, companies need to attract new talents who are increasingly willing to be data-driven.
One of the first challenges for any company at the beginning of its data governance journey is to demonstrate this urgency and convince internal stakeholders of the value of concretely launching a data governance programme.
In this first report, we’ve presented the data governance journey “by the book”. It’s the introductory content in a series that aims to tackle the operational aspects of implementing data governance in the field. Our next article will address the complex bridge between your data governance strategy and the operational need of a use case.