Advancements in AI technology have revolutionised the way we manage and maintain property “hard” assets.
An emerging application is the Intelligent Asset Register, which optimises the management of critical assets such as lifts, electric gates, alarms, and various machinery across extensive property portfolios.
The Challenge
Managing a large portfolio of assets is inherently complex and costly. Consider a large housing association overseeing hundreds of thousands of homes in London and the South East. They manage a vast array of assets, including several hundred lifts, boilers, electric gates and water systems. Their annual expenditure on lifts alone can be substantial, reaching millions of pounds.
For such organisations, the complexity of managing lifts extends beyond financial considerations. The variety of lift models in place means different maintenance schedules and service requirements. Siemens lifts, for example, may offer high reliability and long-term service contracts but come at a higher initial cost. On the other hand, cheaper models from Asian manufacturers might have lower upfront costs but incur higher rates of malfunctions, maintenance expenses and frequent repairs, leading to unexpected downtime. During the planning and development phase of constructions, such matters are very often overlooked or not provided due consideration or consistency of it, which is a large contributing factor to the challenge.
Furthermore, the lift manufacturers also differ with respect to the degree of openness of a service agreement. There are those like KONE and Otis that tend to prefer proprietary servicing agreements, and then there are lower cost lifts that are generally indifferent to who services their lifts e.g. Korean manufacturer Sigma. With such diversity inherent in the system, current manual asset management approaches are inefficient, leading to elevated costs and suboptimal asset performance.
This situation is exacerbated by the need for timely and accurate information to make more informed decisions. For example, suppose a lift frequently breaks down in a high-rise building where elderly or disabled residents live. In that case, it is not just an inconvenience but a critical issue affecting residents’ well-being and safety. The psychological impact of being ‘trapped’ due to an unreliable lift can lead to significant distress and potential mental health issues, especially in social housing or large apartment blocks.
Additionally, ensuring compliance with safety regulations and necessary legal standards is a major concern of real estate organisations. The existence of numerous and diverse building assets (HVAC systems (Heating, Ventilation, and Air Conditioning), lifts, security systems, etc.) across multiple locations and real estate types can mean that keeping track of regulatory compliance, service histories, and maintenance schedules becomes a daunting task. This often results in reactive rather than proactive maintenance.
The Solution: Artefact’s Intelligent Asset Register
Artefact has developed the Intelligent Asset Register to address these challenges. This predictive tool recommends the most suitable asset for the given specifications of a building based on the past performance of client-owned assets.
One significant advantage of modern assets, such as lifts installed in the last decade, is their capability to automatically capture and log a wide range of operational data. These assets are equipped with advanced sensors and monitoring systems that record detailed usage statistics, including the number of trips, load weights, specific floor stops, waiting times, and more. This data is continuously collected and stored in onboard or cloud-based databases, eliminating the need for manual data entry and significantly reducing the potential for human error.
Therefore, the solution makes use of this automatically logged data, alongside other data sources such as past repair bills, lift specifications, and upfront and servicing costs to determine (i.e. predict) what lift(s) give the best asset ROI over time and why, in order to best balance out the initial cost of buying the asset and the servicing and maintenance costs.
The solution works as follows:
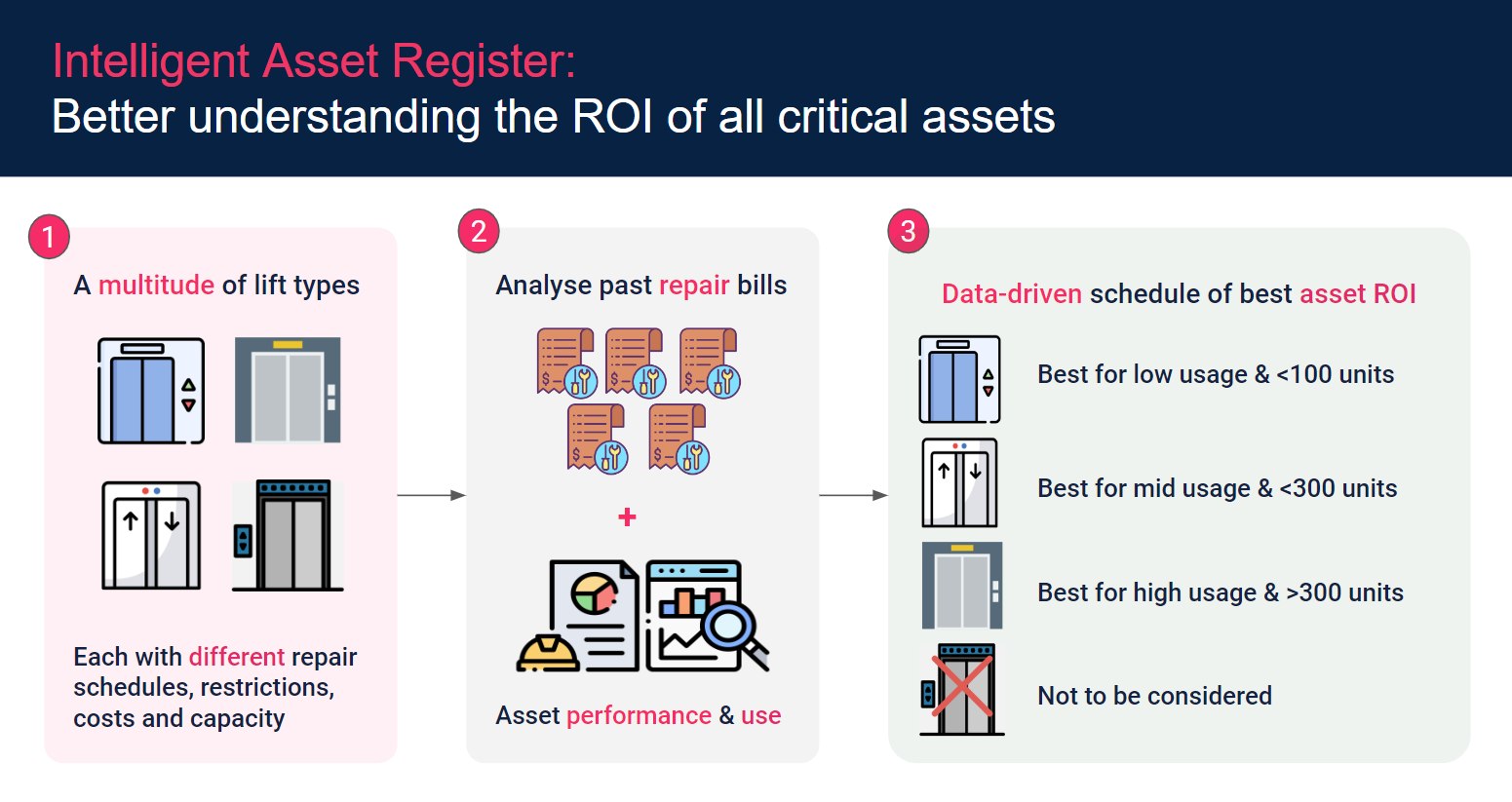
Key benefits
The implementation of the Intelligent Asset Register brings several significant benefits. First and foremost, it offers rapid access to crucial insights, enabling decision-makers to optimise asset choices promptly. Its scalability ensures continuous data ingestion and analysis, aiding in future-proofing efforts as asset portfolios expand.
This AI-driven approach reduces reliance on manual processes, minimising dependency on spreadsheets and key personnel. It streamlines the asset type list for a developer with fewer asset types yielding reduced complexity of management, increased economies of scale with respect to repair bills and increased familiarity with specific models. This all enables improved data collection internally and accelerates the insight generation process, allowing executives to focus on strategic decisions and future recommendations rather than data collection and analysis.
Beyond property management, the Intelligent Asset Register can be applied in various sectors:
As such, we see the following general benefits across industries:
Ensuring Accuracy & Reliability
To ensure the accuracy and reliability of a supervised learning tool designed to recommend assets and provide insights, the following steps are taken:
Recognising Limitations
Complexity: One significant challenge is the need for substantial amounts of high-quality data to train an effective model. If a client’s data is not digitised, the information must first be extracted from handwritten documents and bills using OCR (Optical Character Recognition). This process can be particularly challenging and prone to inaccuracies if the documents have unconventional or complex tabulated formats. In cases where OCR is not feasible, manual data entry and digitisation would be required, which can be time-consuming and resource-intensive.
Historical Bias: The tool’s recommendations are limited to assets that have been used in the past. Consequently, it cannot provide insights on new or recently introduced assets due to insufficient long-term data. This constraint means that new models and innovations might not be included in the recommendations, potentially leading to suggestions of outdated assets that have been superseded by more advanced options. However, one way to overcome this limitation is by extrapolating data for new models that are simply upgraded versions of existing models from the same manufacturer.
Costs and requirements
To build an effective asset recommendation tool, we require comprehensive data, including detailed operational data such as usage statistics, maintenance records, and asset performance metrics, as well as financial data covering initial costs, historical maintenance expenses, and projected costs for new assets. Additionally, we need specifications of existing and past assets, along with any relevant building information about where these assets are or have been in use. Accordingly, deployment costs of Artefact’s Intelligent Asset Register are highly dependent upon the exam question to answer, the quality of available data and the scope of the project, to include the number and different types of assets. As such, the costs of the intelligent asset register solution would reasonably start from c.£70k upwards.
Furthermore, successful deployment of the solution requires close collaboration with key stakeholders and industry experts alike to help validate the model’s recommendations and to ensure alignment with best practices and regulatory standards. In order to facilitate the tool’s successful adoption and continuous improvement, we are able to support clients with user training and the establishment of a feedback mechanism. This helps to ensure our solution can meet the evolving needs of clients effectively.
Why Artefact?
Artefact is a leading global consulting company dedicated to accelerating the adoption of data and AI to positively impact people and organisations. We specialise in data transformation and data marketing to drive tangible business results across the entire enterprise value chain. Artefact offers the most comprehensive set of data-driven solutions, built on deep data science and cutting-edge AI technologies, delivering AI projects at scale across the property sector in the UK.
We are trusted partners to property businesses across residential, commercial, industrial and specialist asset classes. Our partners include FTSE 350 listed companies and similar-sized private organisations. With over 20 years of experience in real estate, our dedicated property team includes experts and chartered professionals in property valuations, urban planning, development, and financing.
Our previous work ranges from developing data-led dynamic strategies with our clients – informing them where to play and how to win in their chosen markets – to major operational changes, such as establishing new business arms and propositions. We have worked in every stage of the property lifecycle, from land acquisition to ongoing maintenance, and have worked with clients to scientifically improve these processes.