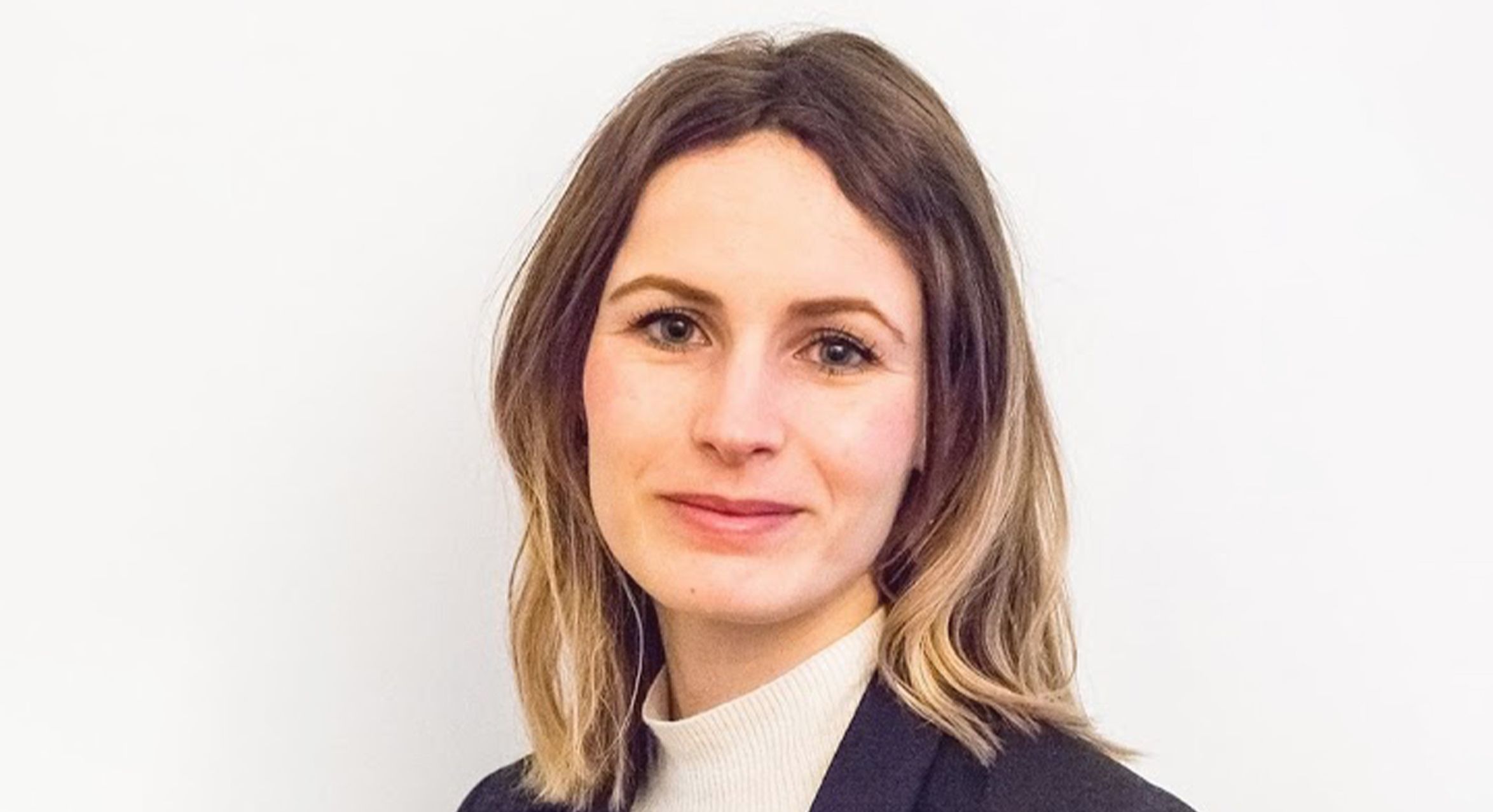
NEWS / DATA CONSULTING
28 October 2020
Data platforms have revolutionised how brands store, analyse and use data — but to use them more efficiently they need to start embedding data governance as code, writes Justine Nerce, Data Consulting Director, and Jean-Baptiste Charruey, Manager Data Engineering, at Artefact.
As global economies begin to recover from the initial shock of coronavirus, we can expect a period of consolidation and re-evaluation by businesses. However, the need for innovation is going nowhere even while budgets may be tight. The launch of new products and services still accounts for over 25% of total revenue and profits.
Innovation needs to be guided by accurate, high-quality data. However, for this to be possible, companies need a foundation of easily accessible, documented and standardised data to draw from. Development cycles for new products and services are becoming shorter and more competitive, so organisations need to evolve their approach to data in order to keep up.
The rise of the data platform has served companies well in accelerating access to data, especially those looking to build the next generation of AI solutions. However, it’s clear brands now need a more robust, efficient and qualitative approach to make their data platforms case agnostic – maintainable, operational and scalable for any cloud, on-prem or hybrid infrastructure.
The rise and fall of the data platform
Businesses constantly revolutionise their approach to data to gain market advantage. Over the decades, data warehouses – large repositories of filtered data – have given way to data lakes – vast, centralised stores of unrefined raw data. Yet, these huge stores of data have proven unwieldy and difficult to govern. Lead times were lengthened as there was no clear, agile process in place to streamline development.
As a consequence, what we’re seeing is the movement from the monolithic environments of old to a more distributed data architecture, based on multiple data platforms. These are sets of software and services that surround a data lake to help make the data more exploitable. Organisations are often building multiple data platforms for each business domain and for every new project. This provides development teams with fast access to the data and insights they need to create new business value that respond to their current needs.
However, with decentralisation comes fragmentation and duplication. Many companies devote massive amounts of time and resources to constructing a data platform for a particular environment. They then have to do it all over again for the next project or use case, with significant discrepancies depending on the team’s technical knowledge. Costs are multiplied several times over as teams essentially start from scratch every time a new project begins.
So much of the most valuable work companies are doing today – including around artificial intelligence – are cross-department and cross-domain. High-quality data has to be shared between teams and different data platforms to realise its full potential, but how do you maintain quality when data is subjected to a gamut of conflicting policies? A compromise needs to be found between giving teams the local ownership of data to customise and create, and the standardisation of approach to build a solid technology base.
Enter the data mesh
Without some connecting tissue between the different domains, data platforms will fail to deliver the quality data and cost efficiency brands need for fast development. Fortunately, they have a way to evolve their approach. They should evolve their data architecture from a disparate collection of data platforms into what Zhamak Dehghani defines as a ‘data mesh’.
A data mesh is an architecture where distributed data platforms, owned by independent cross-functional teams, are connected via a ‘mesh’ of common policies, governance and tools. This approach brings flexibility and resilience to data platforms by setting a shared base, while also giving teams the freedom to customise their own domain.
This approach turns a data platform from a one-and-done project into a long-term asset, eliminating the duplication of work and the needless drain of resources. However, the drawback of the data mesh is that individual teams have to do a lot of work to ensure the industrialisation has been completed. This may be time-consuming with a result that’s far from perfect. Having a template that handles all requirements to make a production-ready solution is key. Yet, what form should this template take?
The main component is a set of common codes that sit across all data platforms. This ‘data sentinel’ is a mix of solutions that facilitate the treatment and analysis of the data and the transition to industrialisation. Its role is to supervise and streamline all data flows – such as the collection of metadata and cleansing – through the development of modules around data quality and documentation.
A data sentinel frees up data teams and specialists from the mundane and repetitive chores of data management. Instead, they can focus on more strategic and innovative tasks that create new value for the business.
At the core of data sentinel, data governance as code should be firmly embedded into platform design and carried on with each new use case. Thanks to data governance as code, data is from the very beginning “owned”, of high quality, documented, secured and compliant, as well as easily accessible through data models across the organisation.
Making innovation ordinary
Data platforms should be evolving products, meant for data activation and fast business value. When mutualised across different use cases and requirements, they make innovation and invention faster and more cost-effective. Indeed, service mutualisation can cut implementation velocity by 40%, helping departments generate value by offering the data quality and variety needed for their use cases.
Businesses have a constant stream of new use cases and products to develop, especially in the current climate. A mutualised, data governance as code approach provides an end-to-end process where they can truly industrialise these use cases. High-quality, accurate data can easily be shared between projects and teams through a robust, highly templatised solution. No time is wasted whenever insight is needed for a new product.
Technology alone is not enough. To make the data platform work, you need to take an approach that’s iterative and transversal. It’s the only way to make innovation ordinary at your company.
First published by ITPortal.com