Not long ago, the term “digital twin” was still just an academic concept. But today, digital twins are found in almost every industry: manufacturing, retail, energy, healthcare and more… bringing benefits including increased productivity, reduced time to market, and improved customer satisfaction. In fact, any use case related to data-driven insights and decision making in today’s interconnected world of physical objects, processes or systems is related to the term digital twin.
Broadly speaking, digital twins are virtual representations of objects (a turbine, a car, a person), processes (supply chain or manufacturing line), or entire systems (a power grid, a hospital, a city). The common denominator? Their underlying data refers to physical and real-world measurements.
What exactly are digital twins? What do they do?
Today, digital twins are making their mark in every corner of industry, from manufacturing to retail, automotive, energy, healthcare, cities, research… the list goes on. Use cases and applications for digital twins are even broader: data monitoring and visualization, efficiency improvement, optimization, scenario analysis, simulation, future state prediction. With such a wide range of industries and applications, it’s not surprising that the term “digital twin” lacks a clear definition and can mean different things to different people.
In this article, we unpack the key characteristics of digital twins to help you better understand and navigate future conversations. We also explain the differences between the two archetypes of digital twins, each of which is motivated by different data requirements, modeling efforts, team skills, and use cases.
Armed with this knowledge, you’ll be able to more easily identify and reap the benefits of this concept for your specific industry and use case.
Digital twin Archetype A: Visualization and analytics in a data twin
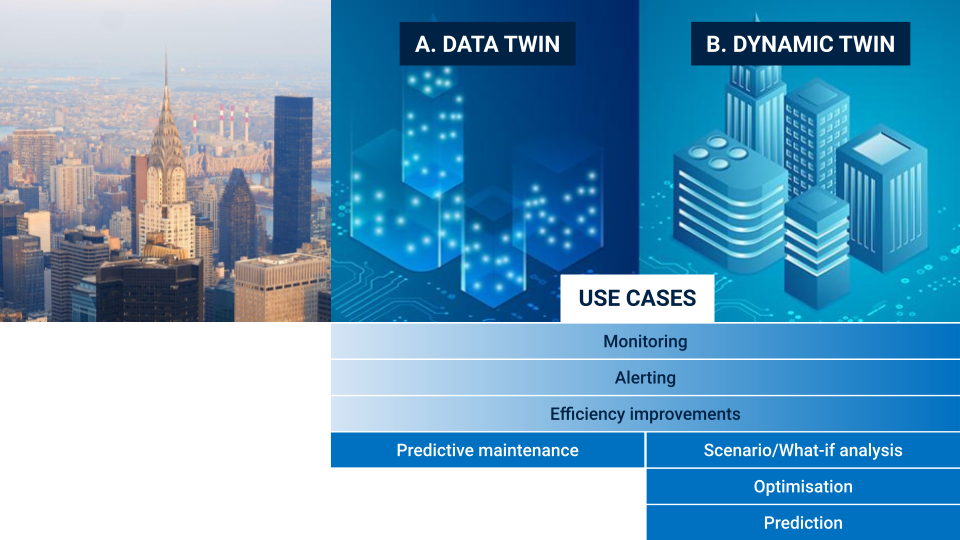
Archetype A visualizes ingested data and provides insights by analyzing measured data. In other industries and processes, this archetype could more generally be described as business intelligence, with the nuance that the digital twin usually visualizes live data. Some companies refer to this archetype as a “data twin.”
The main benefit of a data twin is the availability of cleaned data in a single location, making it accessible to a wide audience to provide a holistic view of the object, process, or system in question. Transparency and access to clean data, without AI or advanced machine learning algorithms, still provides a wealth of information and substantial value to users. Additional value is generated by use cases like monitoring, issue detection and alerting, and adding human interaction and feedback like task management or ticket creation makes the data twin part of an integrated workflow management system. More advanced applications use the incoming data to provide predictive maintenance insights, determining when a device or machine needs to be serviced and adjusting the maintenance cycle based on the measured data.
Examples of a visualization and analytics data twin:
The main challenges in creating a high-impact data twin lie in combining the user’s business knowledge and technological expertise to create the data twin. With cleaned and categorized input data as described in the previous step, the key success factor is to start from the user perspective with a user-centric design approach. Based on clearly defined user stories, we design the graphical interface and derive the technical requirements for data availability. Striking the right balance between visualizing the minimum required data and providing a complete end-to-end picture provides the right level of clarity that creates insight and value.
Digital twin Archetype B: Simulations through a dynamic twin
Archetype B, the “dynamic” digital twin, creates a complete digital representation of reality by dynamically generating information and data in all previously unmeasured locations. The dynamic digital twin takes data available in the data twin and generates additional information to create a holistic end-to-end view of the entire object, system or process.
At the heart of a dynamic digital twin are computation methods that can fill in the blanks where there is no data available. Methods for generating additional data are diverse, ranging from models based on physical equations that capture the full dynamics of the system in detail, to simplified heuristics, statistical relationships such as parameterizations, or advanced AI and machine learning approaches. These methods are not only used to fill in the blanks in the current state, but are the powerful engine that dynamically generates alternative state outputs used for scenario analysis and optimization as described in the use cases section below.
The greatest value of dynamic digital twins lies in using the ability to use their dynamic computation engine to explore and understand how the asset, process or system responds to changes. Running alternative scenarios, performing what-if analyses, and understanding the impact of these changes on the system are powerful use cases for improving product design, optimizing operations, enhancing planning, and ensuring supply security. A few examples:
While the benefits of dynamic digital twins are undisputed, the key challenge for a dynamic digital twin comes from the different methods that are the core computation engine of the digital twin. In many cases a simple heuristic or parameterization will be the best solution; in other cases, more advanced statistical techniques such as machine learning will be more appropriate. The most accurate method remains the use of physical equations to fill in the blanks and determine how the system will react to changes. Criteria for assessing which method is the right choice will depend on the availability of data for training and deployment of models, use cases, level of output accuracy, and computational power for live calculations.
How to get started with a digital twin
One of the biggest challenges in realizing the value of digital twins is to translate a very broad concept into practice to create value through a specific use case. Three aspects are critical in the early stages of the digital twin journey:
While the benefits of dynamic digital twins are undisputed, the key challenge for a dynamic digital twin comes from the different methods that are the core computation engine of the digital twin. In many cases a simple heuristic or parameterization will be the best solution; in other cases, more advanced statistical techniques such as machine learning will be more appropriate. The most accurate method remains the use of physical equations to fill in the blanks and determine how the system will react to changes. Criteria for assessing which method is the right choice will depend on the availability of data for training and deployment of models, use cases, level of output accuracy, and computational power for live calculations.
To summarize: Create value from physical data through digital twins
Digital twins have evolved from an academic concept to a transformative tool that has been successfully deployed across various industries. In today’s interconnected world of physical measurements, digital twins provide virtual representations of physical objects, processes, or systems to enable data-driven insights and decision-making. With two main archetypes, (A) data twins for visualization and analytics, and (B) dynamic twins for simulations and scenario analysis, digital twins address diverse applications and industries. As businesses identify specific use cases, they can ensure a competitive edge by leveraging digital twins to increase productivity, optimize operations, and improve planning.